RDD Operations
The RDD provides the two types of operations:
Transformation
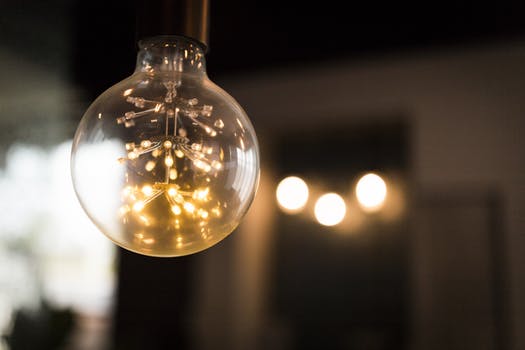
In Spark, the role of transformation is to create a new dataset from an existing one. The transformations are considered lazy as they only computed when an action requires a result to be returned to the driver program.
Let's see some of the frequently used RDD Transformations.
Transformation |
Description |
map(func) |
It returns a new distributed dataset formed by passing each element of the source through a function func. |
filter(func) |
It returns a new dataset formed by selecting those elements of the source on which func returns true. |
flatMap(func) |
Here, each input item can be mapped to zero or more output items, so func should return a sequence rather than a single item. |
mapPartitions(func) |
It is similar to map, but runs separately on each partition (block) of the RDD, so func must be of type Iterator<T> => Iterator<U> when running on an RDD of type T. |
mapPartitionsWithIndex(func) |
It is similar to mapPartitions that provides func with an integer value representing the index of the partition, so func must be of type (Int, Iterator<T>) => Iterator<U> when running on an RDD of type T. |
sample(withReplacement, fraction, seed) |
It samples the fraction fraction of the data, with or without replacement, using a given random number generator seed. |
union(otherDataset) |
It returns a new dataset that contains the union of the elements in the source dataset and the argument. |
intersection(otherDataset) |
It returns a new RDD that contains the intersection of elements in the source dataset and the argument. |
distinct([numPartitions])) |
It returns a new dataset that contains the distinct elements of the source dataset. |
groupByKey([numPartitions]) |
It returns a dataset of (K, Iterable) pairs when called on a dataset of (K, V) pairs. |
reduceByKey(func, [numPartitions]) |
When called on a dataset of (K, V) pairs, returns a dataset of (K, V) pairs where the values for each key are aggregated using the given reduce function func, which must be of type (V,V) => V. |
aggregateByKey(zeroValue)(seqOp, combOp, [numPartitions]) |
When called on a dataset of (K, V) pairs, returns a dataset of (K, U) pairs where the values for each key are aggregated using the given combine functions and a neutral "zero" value. |
sortByKey([ascending], [numPartitions]) |
It returns a dataset of key-value pairs sorted by keys in ascending or descending order, as specified in the boolean ascending argument. |
join(otherDataset, [numPartitions]) |
When called on datasets of type (K, V) and (K, W), returns a dataset of (K, (V, W)) pairs with all pairs of elements for each key. Outer joins are supported through leftOuterJoin, rightOuterJoin, and fullOuterJoin. |
cogroup(otherDataset, [numPartitions]) |
When called on datasets of type (K, V) and (K, W), returns a dataset of (K, (Iterable, Iterable)) tuples. This operation is also called groupWith. |
cartesian(otherDataset) |
When called on datasets of types T and U, returns a dataset of (T, U) pairs (all pairs of elements). |
pipe(command, [envVars]) |
Pipe each partition of the RDD through a shell command, e.g. a Perl or bash script. |
coalesce(numPartitions) |
It decreases the number of partitions in the RDD to numPartitions. |
repartition(numPartitions) |
It reshuffles the data in the RDD randomly to create either more or fewer partitions and balance it across them. |
repartitionAndSortWithinPartitions(partitioner) |
It repartition the RDD according to the given partitioner and, within each resulting partition, sort records by their keys. |
Action
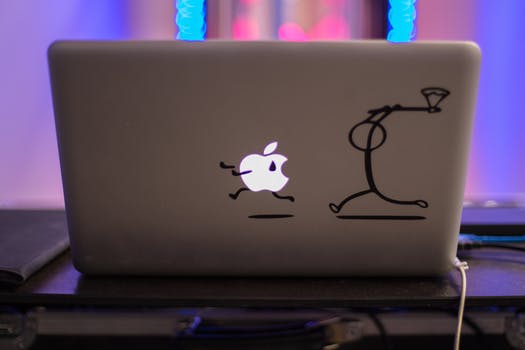
In Spark, the role of action is to return a value to the driver program after running a computation on the dataset.
Let's see some of the frequently used RDD Actions.
Action |
Description |
reduce(func) |
It aggregate the elements of the dataset using a function func (which takes two arguments and returns one). The function should be commutative and associative so that it can be computed correctly in parallel. |
collect() |
It returns all the elements of the dataset as an array at the driver program. This is usually useful after a filter or other operation that returns a sufficiently small subset of the data. |
count() |
It returns the number of elements in the dataset. |
first() |
It returns the first element of the dataset (similar to take(1)). |
take(n) |
It returns an array with the first n elements of the dataset. |
takeSample(withReplacement, num, [seed]) |
It returns an array with a random sample of num elements of the dataset, with or without replacement, optionally pre-specifying a random number generator seed. |
takeOrdered(n, [ordering]) |
It returns the first n elements of the RDD using either their natural order or a custom comparator. |
saveAsTextFile(path) |
It is used to write the elements of the dataset as a text file (or set of text files) in a given directory in the local filesystem, HDFS or any other Hadoop-supported file system. Spark calls toString on each element to convert it to a line of text in the file. |
saveAsSequenceFile(path)
(Java and Scala) |
It is used to write the elements of the dataset as a Hadoop SequenceFile in a given path in the local filesystem, HDFS or any other Hadoop-supported file system. |
saveAsObjectFile(path)
(Java and Scala) |
It is used to write the elements of the dataset in a simple format using Java serialization, which can then be loaded usingSparkContext.objectFile(). |
countByKey() |
It is only available on RDDs of type (K, V). Thus, it returns a hashmap of (K, Int) pairs with the count of each key. |
foreach(func) |
It runs a function func on each element of the dataset for side effects such as updating an Accumulator or interacting with external storage systems. |
|